Soft materials are ubiquitous in everyday life, including products such as food and cosmetics, but also biological and bioinspried materials in general. Chraracterise by energy scales that are influenced by thermal motion, they show complex features over a wide range of length and time scales. Computational soft matter research aims to understand and predicted the emergent complexity of soft-matter systems by means of simulations, theory and modelling. This interdisciplinary discipline requires a combination of advanced computational methods, theoretical physics and chemistry, as well as more recent data-driven methods. The computational soft matter laboratory has been recently founded to simulate the interdisciplinary effort .
Self assembly
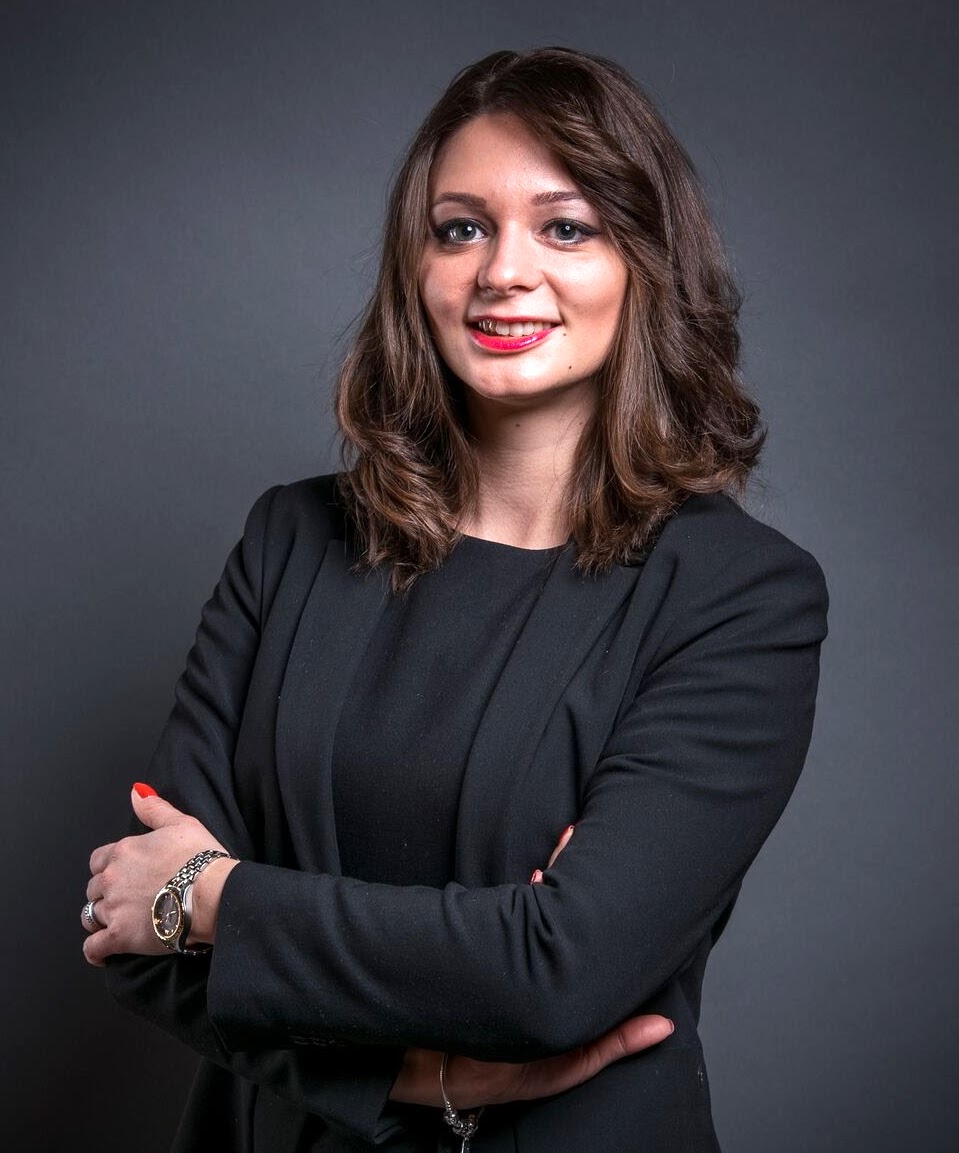
Dr. Ir. Ioana M. ILIE
Computational Chemistry Group.
The self-assembly of proteins into ordered aggregates on relevant timescales is computationally very demanding at atomistic resolution. Hence, the thermodynamics and kinetics of aggregate formation are difficult to be explored. Understanding the accumulation of proteins and their relationship to triggering various biological responses are crucial elements in generating reliable predictions and explaining experimental observations.
Patchy particles are well suited to model self-assembly of proteins into ordered or amorphous aggregates. Combined with Brownian dynamics they provide insight into the dynamics of the assembly process. Proteins are modelled as single nanometer sized particles decorated with interaction patches. Additionally, the particles are able to change shape and interaction parameters in response to the environment. In collaboration with the Organic Chemistry and Physical Chemistry group at the Department of Biomolecular Sciences of Wageningen University, we explore the aggregation of polypeptides associated with neurodegenerative diseases (e.g. amyloid-beta for Alzheimer’s disease, alpha-synuclein for Parkinson’s disease) by developing novel models and using advances simulation methods.
Active Soft Matter
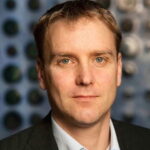
Prof. dr. Peter Bolhuis
Computational Chemistry Group.
Structural architectures in living cells, such as the cytoskeleton in muscle or plant tissue, are both viscoelastic and active, i.e. undergo continuous injection of energy, leading to remarkable collective, non-equilibrium properties. The thermodynamics, kinetics and statistical mechanics of such systems are far from understood. While active fluids (swarms, swimmers) have seen an explosion of interest recently, their solid (elastic) counterparts remain completely unexplored and understanding such phenomena remains one of the grand challenges of modern statistical physics.
Patchy particles provide a model system for exploring complex molecular structures with high controllability. Patchy particles are micron-sized colloidal particles dressed with patches that are able to form bonds under specific solvent compositions and temperatures. While patchy particles are big enough to be observed in experiment under e.g. a confocal microscope, they are small enough to exhibit similar statistical behavior as atoms and molecules. In addition, patchy particles are a mesoscopic structural analogue of carbon atoms. Divalent patchy particles make linear bonds like sp hybridized carbon atoms and act as monomers forming chains as shown in the background. Tetravalent patchy particles make tetrahedral bonds and may form rings with a half-chair or envelope conformation like cyclopentane. In collaboration with the experimental Soft Matter group we explore active patchy particle architectures, by using advanced simulation methods, including path sampling.
Design of soft matter

Dr. Alberto Pérez de Alba Ortíz
Computational Soft Matter & Chemistry
Alberto Pérez de Alba Ortíz focuses on the development of computational frameworks for bottom-up design of self-assembling materials with tailor-made properties. To this end, his group uses evolutionary, Bayesian and active learning models to predict desirable properties and thus decide which combination of design parameters is more suitable to try next, either in simulation or experiments. The data collected during these iterative design also serves as training for future generative and predictive machine learning models. Current projects are focused on time-dependent protocols for colloidal self-assembly and responsive mechanical properties in polymers, as well as self-assembly of two-component quasicrystals and zeolitic phases. More over, by means of mimicking indirect observations, these design strategies can explain measurements of matter that is impossible to reach by experiments, e.g., the phase behavior of iron at our planet’s inner-core.