The striking fact about many, and likely all, living systems is that they display robust, ordered and anticipatory (‘smart’) behavior in spite of inherent stochasticity. When transitioning to the living state, these systems do not leave randomness behind, which makes their behavior all the more difficult to understand and to emulate in artificial systems and models. It is currently poorly understood how to characterize, create or model, the energy-driven pathways out of the random and into the ordered state, or how to access such non-equilibrium states through energy-driven disorder-order transitions (Fig. 1). This means that our understanding of out-of-equilibrium systems, emergence, and life, is still incomplete.
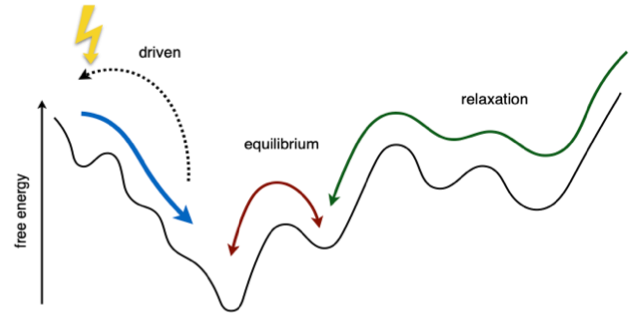
One of the formidable challenges in this field is unravelling the emergence of autocatalytic (self-replicating) systems, i.e. systems in which entities (molecules, colloidal building blocks, etc.) are able to catalyze their own formation, a basic requirement for a gradual transition from non-living to living systems. Such emergence must include mechanisms that lead to the self-organization of reaction pathways. Understanding how the interactions between the constituents and with the environment shape the overall outcome of the reaction systems might explain how, in the absence of evolution, the prebiotic world was enriched in certain compounds and systems needed for life to emerge.
In this project we use simple (patchy particle) models to create a catalytic cycle, and optimize the (many) parameters for optimal properties (e.g. turnover or response). The basic kinetic steps in any network are diffusion, association, dissociation, and reaction, for which the all-important rate constants can be obtained using rare event trajectory techniques. To optimize the catalysis for a complex network, the number of parameters is large, and a trial and error parameter search is not effective. Using a novel path reweighting scheme it is possible to solve this problem by efficiently computing in which direction the system needs to evolve for improving the catalytic properties. For models with many parameters to optimize, the path reweighting method uses Machine Learning-like protocols to make these predictions and optimization.